RUL, or Remaining Useful Life, is a critical concept in predictive maintenance and reliability engineering. It refers to the estimated lifespan of a component or system before it requires replacement or repair. As industries increasingly adopt data-driven strategies, understanding RUL has become more important than ever before. Whether you're in manufacturing, aviation, or energy production, RUL plays a pivotal role in optimizing operational efficiency and reducing downtime.
In today's fast-paced industrial landscape, organizations are under constant pressure to improve productivity while minimizing costs. Predictive maintenance, powered by advanced analytics and artificial intelligence, has emerged as a game-changer. At the heart of this approach lies the concept of RUL, which allows engineers and decision-makers to make informed predictions about the lifespan of critical assets.
This article delves into the intricacies of RUL, exploring its definition, significance, methodologies, and practical applications. By the end of this comprehensive guide, you'll have a thorough understanding of how RUL works and why it's essential for modern businesses. Whether you're a seasoned professional or new to the field, this article provides valuable insights into leveraging RUL for improved operational performance.
Read also:Nicole Cattell A Rising Star In The Entertainment Industry
Table of Contents
- What is RUL?
- Importance of RUL in Modern Industries
- Methodologies for Estimating RUL
- Data Collection for RUL Estimation
- Applications of RUL Across Industries
- Challenges in Implementing RUL
- Technologies Enabling RUL
- Case Studies Demonstrating RUL Success
- Future Trends in RUL
- Conclusion and Call to Action
What is RUL?
RUL, or Remaining Useful Life, is the estimated time a component, machine, or system can continue operating before it requires maintenance, repair, or replacement. This concept is central to predictive maintenance strategies, allowing organizations to anticipate failures before they occur. By leveraging data analytics and machine learning, engineers can make accurate predictions about the lifespan of critical assets.
Definition and Scope
The scope of RUL extends beyond simple time-based predictions. It involves understanding the degradation patterns of assets and correlating them with operational data. This holistic approach ensures that maintenance activities are scheduled optimally, reducing unnecessary downtime and extending the life of equipment.
Key Components of RUL
- Data collection and analysis
- Modeling degradation patterns
- Integration with existing systems
Importance of RUL in Modern Industries
In today's data-driven world, the importance of RUL cannot be overstated. Industries such as manufacturing, aviation, and energy production rely heavily on predictive maintenance to ensure uninterrupted operations. By accurately estimating RUL, organizations can:
- Reduce unplanned downtime
- Optimize maintenance schedules
- Lower operational costs
- Improve asset reliability
According to a study by McKinsey, companies that implement predictive maintenance strategies can reduce maintenance costs by up to 40%. This underscores the significance of RUL in driving operational efficiency.
Read also:Rulz 2022 Exploring The Ultimate Guide To Mastering The Rules Of 2022
Methodologies for Estimating RUL
Several methodologies exist for estimating RUL, each with its own strengths and limitations. The choice of methodology depends on factors such as the type of asset, available data, and organizational goals.
Statistical Models
Statistical models use historical data to predict future performance. These models are effective when large datasets are available and can provide accurate predictions with minimal computational resources.
Machine Learning Approaches
Machine learning algorithms, such as neural networks and decision trees, offer more sophisticated methods for RUL estimation. These approaches can handle complex datasets and identify patterns that may not be apparent through traditional statistical methods.
Data Collection for RUL Estimation
Data collection is a critical step in RUL estimation. High-quality data ensures the accuracy and reliability of predictions. Key considerations include:
- Sensor placement and calibration
- Data preprocessing and cleaning
- Integration with existing systems
Modern technologies, such as the Internet of Things (IoT), have revolutionized data collection by enabling real-time monitoring of assets. This capability enhances the precision of RUL predictions and facilitates timely interventions.
Applications of RUL Across Industries
RUL finds applications in various industries, each with unique challenges and requirements. Below are some examples:
Manufacturing
In manufacturing, RUL helps optimize production schedules and minimize downtime. By predicting the lifespan of machinery, organizations can plan maintenance activities during non-peak hours, ensuring uninterrupted operations.
Aviation
The aviation industry relies heavily on RUL to ensure the safety and reliability of aircraft. Predictive maintenance strategies, powered by RUL, help airlines reduce maintenance costs and improve flight schedules.
Challenges in Implementing RUL
Despite its benefits, implementing RUL poses several challenges. These include:
- Data quality and availability
- Complexity of models
- Integration with existing systems
Overcoming these challenges requires a combination of technical expertise, organizational commitment, and investment in infrastructure.
Technologies Enabling RUL
Advances in technology have significantly enhanced the capabilities of RUL. Key enabling technologies include:
- IoT sensors
- Big data analytics
- Artificial intelligence and machine learning
These technologies work together to provide real-time insights into asset performance, enabling organizations to make data-driven decisions.
Case Studies Demonstrating RUL Success
Several organizations have successfully implemented RUL strategies, achieving significant improvements in operational efficiency. For example:
Case Study 1: Manufacturing Plant
A manufacturing plant implemented RUL-based predictive maintenance, resulting in a 30% reduction in maintenance costs and a 20% increase in equipment uptime.
Case Study 2: Aviation Company
An aviation company adopted RUL to monitor engine performance, leading to a 15% decrease in maintenance-related delays and improved passenger satisfaction.
Future Trends in RUL
The future of RUL looks promising, with advancements in technology and increasing adoption across industries. Key trends include:
- Increased use of AI and machine learning
- Integration with digital twins
- Adoption of edge computing for real-time analysis
As organizations continue to embrace digital transformation, RUL will play an even more critical role in driving operational excellence.
Conclusion and Call to Action
In conclusion, RUL is a vital concept in predictive maintenance and reliability engineering. By accurately estimating the Remaining Useful Life of assets, organizations can optimize maintenance schedules, reduce costs, and improve operational efficiency. The integration of advanced technologies, such as IoT and AI, further enhances the capabilities of RUL, making it an indispensable tool for modern businesses.
We invite you to share your thoughts and experiences with RUL in the comments section below. Additionally, explore our other articles for more insights into predictive maintenance and related topics. Together, let's drive innovation and excellence in the field of industrial operations!
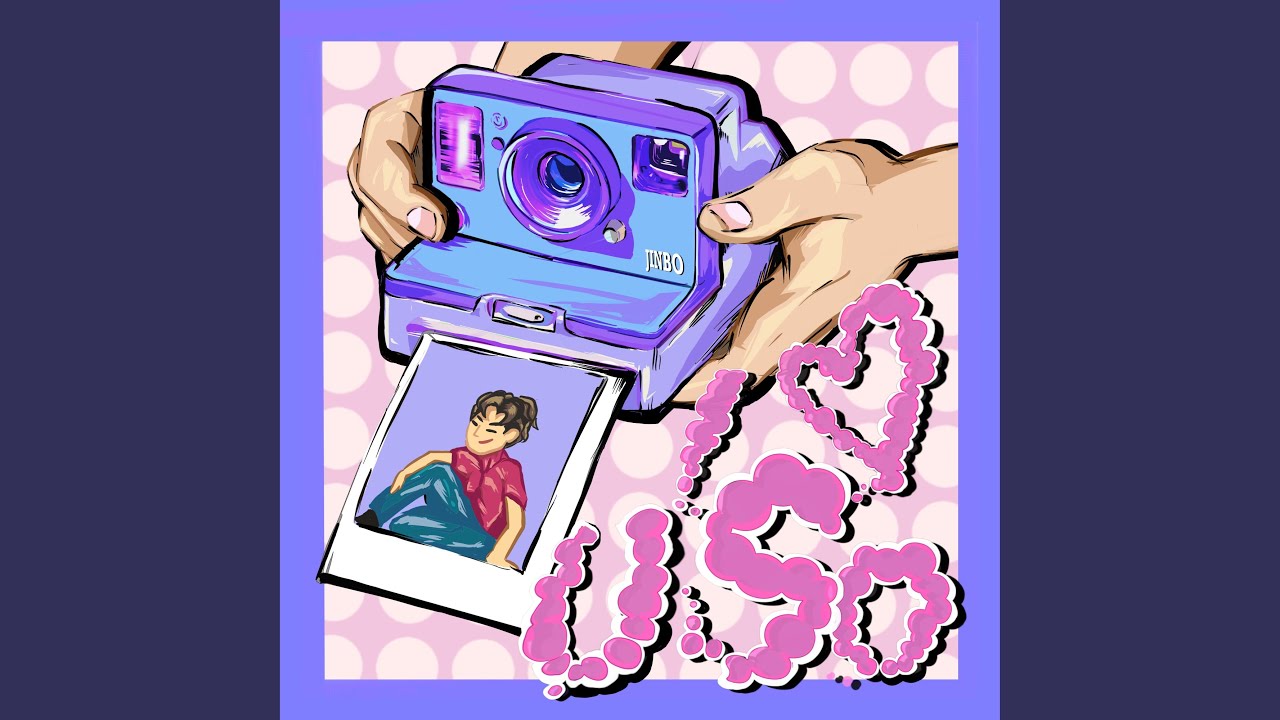

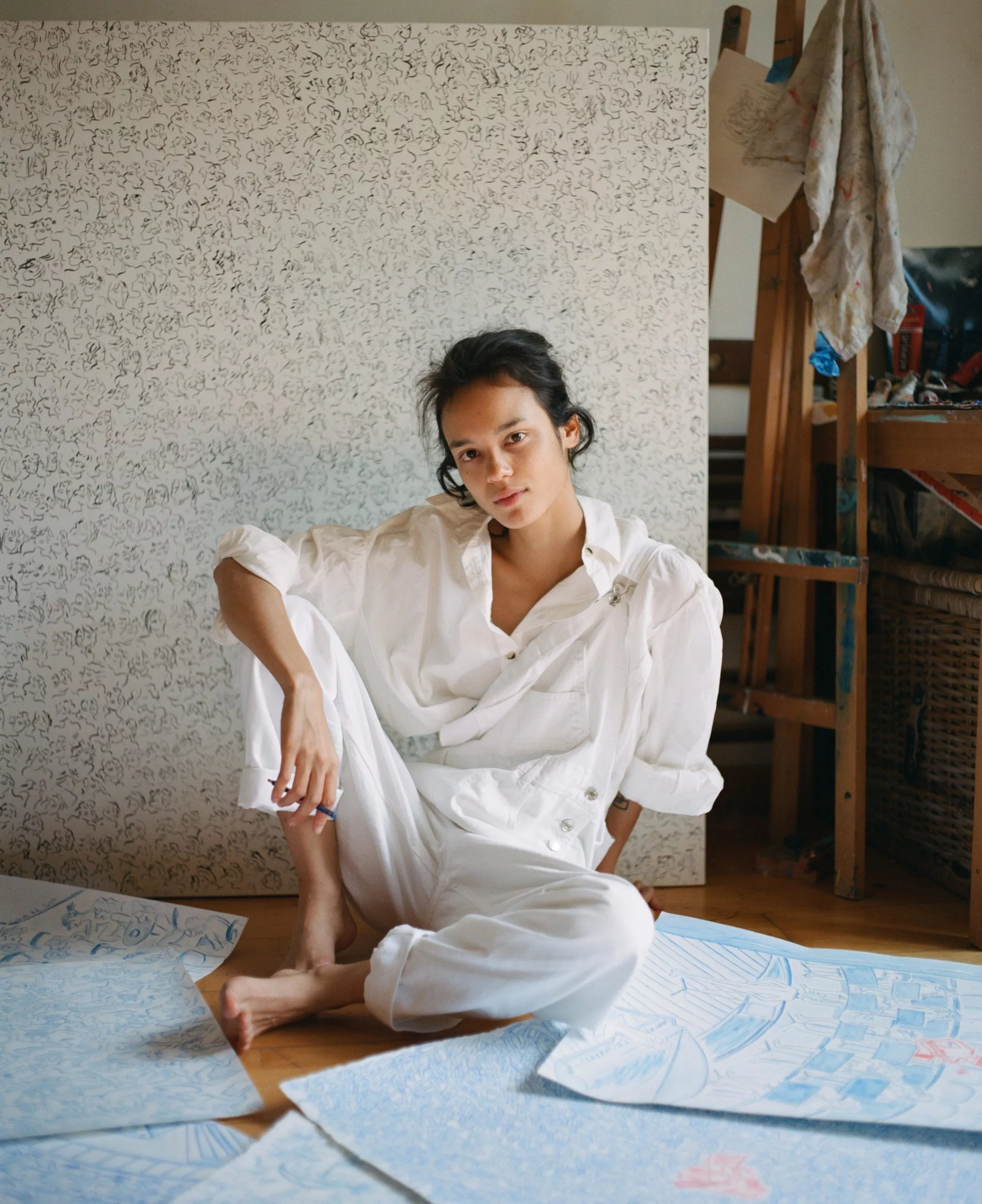